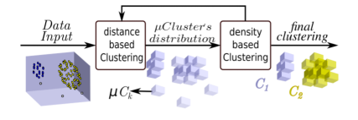
Caractéristiques
- Types de propriété intellectuelle : Logiciel
- Stade de développement : TRL4 - Validation de la technologie en laboratoire
-
Secteurs d'applications :
Numérique - Réseaux - Télécoms - Systèmes
-
Domaines scientifiques :
SCIENCES DE L'INGÉNIEURSCIENCES ET TECHNOLOGIES DE L'INFORMATION ET DE LA COMMUNICATION
- Mots-clés : AI ; Predictive maintenance ; Data analytics
Description
Predictive maintenance relies on clustering algorithms to track unwanted changes in industrial processes and infrastructures. However, most of them tend to fail to detect slow deviations indicating a gradual deterioration of the system.
Monitoring the condition of industrial systems is critical to safety and efficiency. Shocks, vibration, heat, friction or dust for instance can degrade processes behaviors. Yet data science can detect the emergence of anomalies before they cause failure.
Data clustering, or unsupervised learning, looks for undetected patterns in a data set with no pre-existing labels and with a minimum of human supervision. Unusual events can be identified when an unintended pattern arises. But traditional clustering algorithms may fail to detect changes arising slowly over time.
Spécifications techniques
Data |
•Quantitative
•Qualitative e.g. [open, closed]
|
Language |
Python |
System(s) |
Linux, Windows, MacOs |
Avantages concurrentiels
• Dynamic tracking of even the slowest deviations
• Effective cluster detection and good outlier rejection properties
• Simplified configuration with a single parameter
Champs d'application
• Predictive maintenance
• System health diagnosis
• Process monitoring
• Data analytics